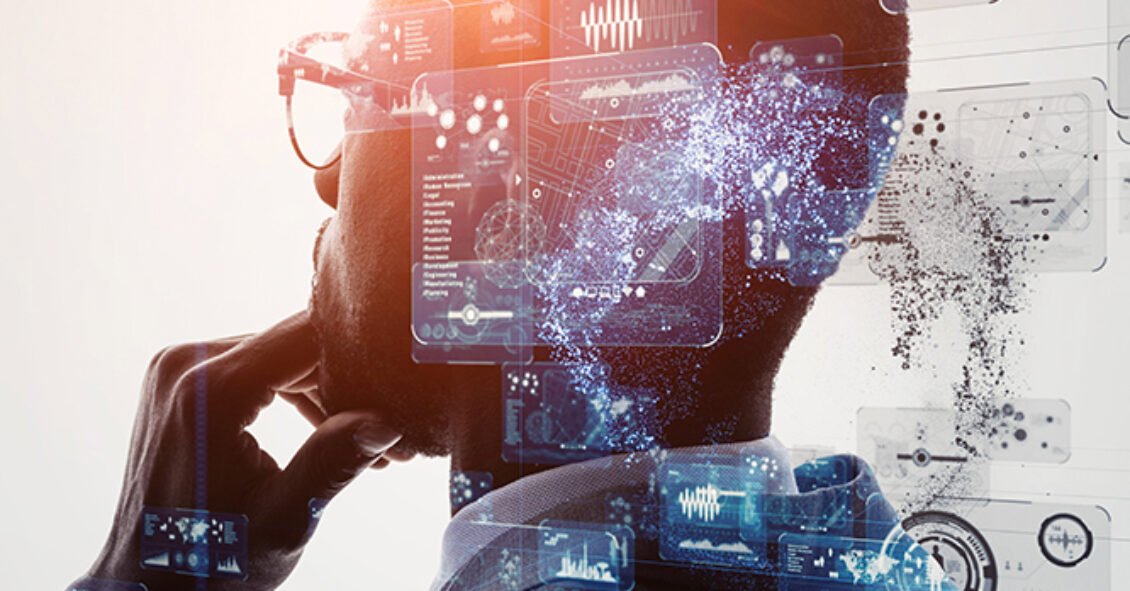
Jensen Huang took the stage at GTC 2025 with his signature leather jacket and a grin that signaled he was about to deliver another industry-defining keynote.
He wasted no time in setting the tone with his characteristic humor: “You know, if you’re still looking to buy a Hopper… don’t be afraid. It’s okay. There are circumstances where Hopper is fine… just not many.”
Reach out to discuss this topic in depth.
Huang’s opening remarks encapsulated the magnitude of change in artificial intelligence (AI) infrastructure. With agentic AI demanding 100x more computation than anticipated, the world’s data centers are racing to keep up. Huang quipped, “The future of software requires capital investment. Your software is no longer just code—it’s a machine generating intelligence.”
Most importantly, Huang emphasized that AI’s shift to reasoning-based architectures dramatically increases computational needs. NVIDIA’s R1 model outperformed standard Large Language Models (LLMs) in structured problem-solving, requiring 8,600 tokens versus 439 tokens for a non-reasoning model. Hence, while AI can now “think” step by step, improving accuracy and logical consistency, running a reasoning model requires significantly more compute cycles.
And with that, he launched into the future of AI infrastructure, reasoning models, and the role of NVIDIA’s Blackwell architecture in the next wave of computing.
Eventually, he wrapped up by focusing robotics and physical AI – the next frontier in AI (to put it cynically, the “necessary” frontier required to keep feeding the beast that NVIDIA has become). Let’s break down the three major announcements I liked.
Key announcements
- Blackwell Architecture and AI factories
NVIDIA unveiled the Blackwell B200 Graphics Processing Unit (GPU), engineered for extreme-scale AI. Featuring MVLink 72 and FP4 precision, Blackwell achieves a 40x performance leap over Hopper in inference workloads. The company introduced Dynamo, an AI factory operating system designed to optimize token generation and maximize efficiency.
Pros:
- Extreme AI scaling: 25x improvement in AI factory efficiency under International Organization for Standardization (ISO) power constraints
- Lower cost per token: The ultimate bottleneck for AI enterprises is energy consumption, and Blackwell optimizes TCO
- Disaggregated MVLink 72: Scaling AI from the chip level to data centers, turning clusters of GPUs into a single compute entity
Cons:
- Increased complexity: The AI factory model requires enterprises to rethink data center architecture
- Massive investment requirement: Companies must heavily invest in liquid-cooled AI infrastructure
- Short hardware cycles: Rapid obsolescence of prior architectures like Hopper could strain Information Technology (IT) budgets
- Expansion into Enterprise AI and AI-Driven storage
Huang introduced a new class of AI workstations—DGX Station and DGX Park—designed to bring AI into enterprise environments. NVIDIA has also announced AI-native storage solutions, embedding Generative AI (gen AI) into enterprise data systems.
Pros:
- AI for every business: Enterprises can now deploy generative AI directly in-house
- Semantic storage revolution: Data retrieval will shift from traditional search to natural language querying
Cons:
- Enterprise adoption challenges: Transitioning from retrieval-based computing to AI-driven storage requires significant software upgrades
- Robotics and general-purpose AI for physical environments
NVIDIA introduced Groot N1, a foundation model for humanoid robots. The company also unveiled Newton, a physics engine co-developed with DeepMind and Disney Research, designed to improve robotic dexterity and real-world adaptability. A particularly striking demonstration featured Blue, a robot co-developed with Disney Research, showcasing lifelike movements and AI-driven responsiveness, hinting at a future where general-purpose robots become an integral part of everyday life.
Pros:
- Standardized AI for robots: A foundation model accelerates humanoid robot development
- Physics-based AI training: Newton enables robots to simulate physical interactions in real-time, vastly improving real-world performance
Cons:
- Still in early adoption: Large-scale deployment of generalist robots remains a long-term vision
- High power requirements: Physical AI demands extreme computation and real-time processing
Comparative analysis: GTC 2025 vs. GTC 2024
Feature | GTC 2024 (Hopper) | GTC 2025 (Blackwell) | Impact |
GPU Performance | 1X (Hopper) | 40X Inference Speed (Blackwell) | Major boost in AI factory efficiency |
AI Model Complexity | Large Language Models (LLMs) | Reasoning AI and Agentic AI | Higher cognitive capabilities |
AI Networking | InfiniBand | Co-packaged Silicon Photonics | Reduced energy overhead for scaled networking |
Digital Twins | Early-stage adoption | Fully integrated Omniverse AI factory planning | Optimized data center build-outs |
Robotics AI | Conceptual models | Open-source Groot N1 for humanoid robots | Accelerated development in robotics |
NVIDIA network effect: Expanding partnerships
NVIDIA’s GTC 2025 keynote reinforced its aspiration to become the backbone of AI transformation, not just through hardware but via strategic partnerships spanning multiple industries.
- One of the most significant collaborations was with T-Mobile, Cisco, and Cerberus, aimed at integrating AI into radio networks, optimizing 5G infrastructure, and making AI-powered edge computing a reality
- In the automotive sector, General Motors (GM) selected NVIDIA as its AI partner to drive advancements in autonomous vehicles, leveraging NVIDIA’s training, simulation, and robotics AI stack
- Meanwhile, BlackRock is working with NVIDIA to harness AI for financial analytics and risk modeling, illustrating the growing influence of AI in finance
- On the cloud front, CoreWeave and other GPU cloud providers are expanding NVIDIA’s reach, ensuring enterprises have access to AI-native infrastructure at scale
- Additionally, Accenture and Amdocs have joined forces with NVIDIA to develop AI-driven solutions for business and telecommunications, further embedding AI into enterprise operations
Key Implications for stakeholders
Enterprises
- AI-driven workflows will become a necessity, not a luxury
- Companies must prepare for a generational shift in computing infrastructure if they want to implement Systems of Action (SoA)
Service providers and cloud vendors
- AI factories reinforce the cloud’s role as the primary AI processing hub
- Network operators must adapt to AI-driven workloads, investing in high-bandwidth, low-latency infrastructure
Investors
- Long-term value lies in ecosystem players that can complement NVIDIA’s AI stack rather than compete on hardware
- The semiconductor arms race continues, with power efficiency emerging as the key differentiator
Everest Group’s take
GTC 2025 confirmed NVIDIA’s dominance in AI infrastructure but also highlighted two things…
- The rising costs, power requirements and complexities of next-gen AI workloads
- Evolution of the ecosystem play for NVIDIA
The announcements—while ambitious—signal a future where AI systems are not just more powerful but also more operationally demanding. Enterprises who look to build Systems of Action (SoA) must take a process-first mentality and balance the opportunities presented by AI reasoning models and infrastructure advancements with the realities of cost, frictionless architectures, and autonomous decisions.
The industry’s real challenge is to make these advancements real for enterprises.
To discuss the takeaways from GTC 2025 and further advancements in AI, please contact Abhishek Singh ([email protected]).