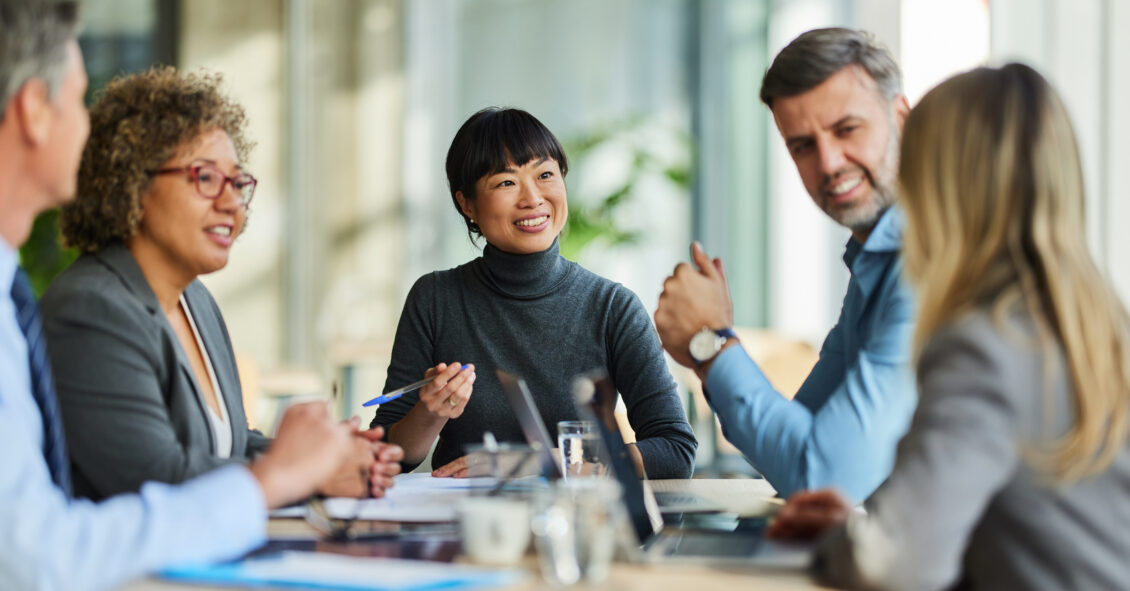
Clinical trials are becoming more complex, diverse, and data-intensive than ever before. With decentralized trials, real-world evidence, and multi-source data streams reshaping the research landscape, effectively managing clinical data is no longer just an operational necessity, it’s a strategic imperative.
At the same time, regulatory agencies are strengthening data standardization requirements, making structured and compliant data crucial for meeting industry demands. But is the Life Sciences industry truly prepared to manage, standardize, and leverage this data to keep studies on track?
Reach out to discuss this topic in depth.
Before exploring Clinical Data Management (CDM), let us first map out the patient journey across various data sources, collection points, and the distinct services at each stage.
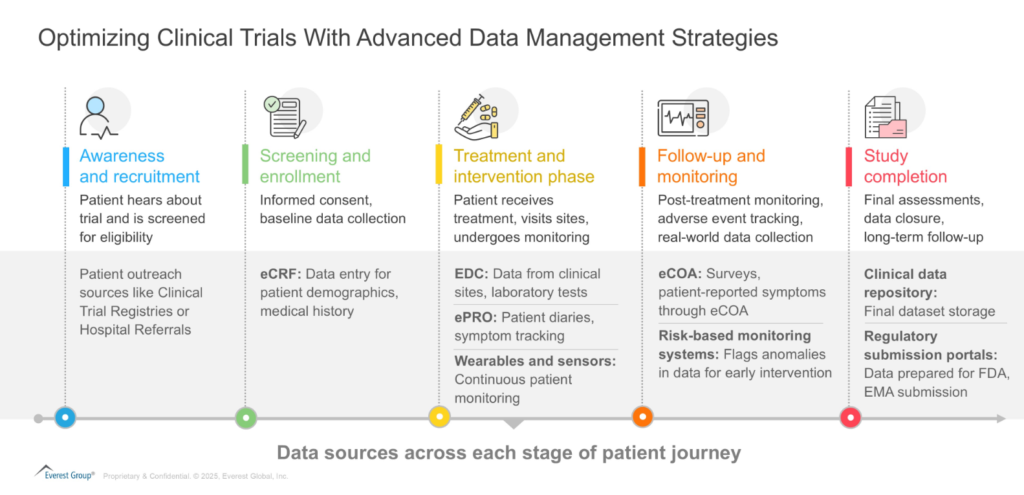
CDM operations encompass the entire lifecycle of clinical trial data, from collection and validation to cleaning, standardization, and the final data lock. It involves designing case report forms (CRFs), setting up Electronic Data Capture (EDC) systems, applying validation rules, and conducting discrepancy management to ensure data integrity. Effective data management throughout the patient journey ensures clinical trials generate reliable, high-quality datasets essential for regulatory submissions.
What is driving the need for next-generation clinical data management solutions now?
Clinical studies collect data from various sources, including Electronic Health Records (EHR), wearable devices, lab systems, and Patient-Reported Outcomes (ePRO/eCOA). However, these data streams vary in format and completeness, posing significant challenges for integration and standardization.
Thus, Next-Generation CDM solutions will leverage advanced technologies such as artificial intelligence (AI), automation, and cloud-based platforms to streamline data collection, processing, and analysis in clinical trials with focus on:
- Harmonizing diverse data streams: CDM standardizes diverse datasets through structured processes, advanced validation techniques, and interoperability standards, ensuring accuracy and consistency
- Enhancing data cleaning and traceability: EDC systems and data transformation tools streamline data cleaning while maintaining traceability throughout the trial lifecycle
- Leveraging AI/Generative AI (gen AI) and automation: AI-driven solutions including anomaly detection and data extraction tools, reduce manual intervention, and improve data accuracy. Additionally, generative AI enhances medical coding, automates query resolution, and enables predictive analytics, accelerating data processing and drug development
However, these advancements also present challenges. The vast and varied data sources create integration and harmonization complexities, requiring strong data governance. Ensuring data security and regulatory compliance is critical, particularly with the growing reliance on cloud-based platforms.
Furthermore, CDM teams must adapt to evolving trial designs, implement AI solutions, and balancing automation with human oversight to maintain data accuracy. As CDM evolves, addressing these challenges will be essential to improve efficiency and enhance trial outcomes.
How are providers adapting to the changing CDM landscape?
Sponsors are increasingly outsourcing CDM functions to Contact Research Organizations (CROs) and specialized providers, leveraging their end-to-end solutions for study start-up, conduct and close-out. CROs such as IQVIA, PPD, and Parexel are investing in AI-driven automation, collaborating with next-generation EDC providers, and integrating real-world data to improve efficiency and streamline operations.
Meanwhile, specialists such as Medpace, Zifo, and Quanticate leverage domain expertise to support complex therapeutics, including cell and gene therapies, precision medicine, and rare diseases.
Information Technology (IT) / Business Process Outsourcing (BPO) firms such as Accenture and Cognizant on the other hand, focus on the technology aspects of CDM, providing scalable solutions for data processing, automation, and integration.
They provide cloud-based platforms, AI-powered analytics, and centralized data management systems that enhance efficiency and reduce manual effort. They also manage high-volume tasks such as data entry, medical coding, query management, and reconciliation.
While they may not have the same therapeutic expertise as CROs, their strength lies in providing scalable, technology-driven solutions that improve data quality, accelerate timelines, and support large-scale clinical programs.
Strategic imperatives for implementing Next-Generation CDM
As clinical trials become more data-intensive, enterprises must adopt strategic approaches to optimize data management and stay ahead of evolving industry demands. Here are four key levers to drive better clinical outcomes:
- Invest in AI-driven automation: Adopt AI-driven tools for real-time data validation, anomaly detection, and automated medical coding to enhance accuracy and efficiency. Leveraging predictive analytics and Natural Language Processing (NLP) for query resolution can further streamline workflows and accelerate trial execution
- Strengthen data governance and standardization: Establish clear governance frameworks and adopt industry standards (e.g., CDISC, HL7 FHIR) to ensure data consistency across diverse sources. Strong governance minimizes discrepancies and accelerates regulatory approvals. Additionally, adhering to guidelines like Good Clinical Data Management Practices (GCDMP) by the Society for Clinical Data Management (SCDM) helps organizations navigate these evolving requirements
- Adopt cloud-based and interoperable platforms: Transition to cloud-based CDM solutions that facilitate real-time data sharing, integration, and scalability. Interoperable platforms improve collaboration across sponsors, CROs, and regulators, streamlining trial execution
- Build cross-functional data expertise: Upskill CDM teams in AI, analytics, and real-world data methodologies. Moreover, enterprises can focus on collaborations between data scientists, regulatory experts, and clinical researchers to enhance decision-making
The future of CDM: A shift to strategic decision making
Clinical Data Management is poised to transition from an operational support function to a strategic enabler of data-driven decision-making in clinical trials. The focus will move beyond data cleaning and validation to proactive insights generation, using advanced analytics for real-time trends and trial optimization.
Greater collaboration between sponsors, regulators, and technology providers will drive the adoption of unified data platforms, ensuring seamless interoperability across global trials.
Additionally, as CDM roles evolve, professionals will require expertise in AI-driven methods and cross-functional data strategies. And as trials grow more complex and patient-centric, CDM will drive faster, adaptive trial designs for better efficiency and outcomes.
If you found this blog interesting, check out our Navigating The Agentic AI Tech Landscape: Discovering The Ideal Strategic Partner | Blog – Everest Group, which delves deeper into another topic regarding AI.
To discuss clinical data management trends in life sciences or other developments in clinical operations space, please contact Vandana Sharma ([email protected]) or Divya Kapoor ([email protected]).