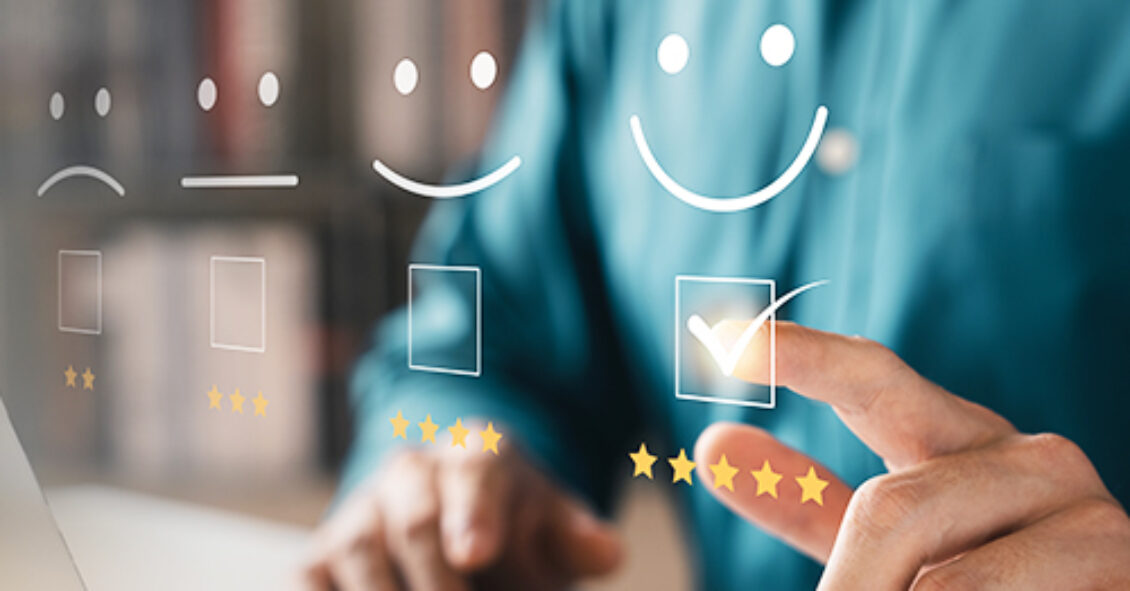
NVIDIA’s recent launch of the Isaac GROOT N1 foundational model for robotics has set the stage for the next revolution in robotic technology, paving the way for a new generation of transformative innovations.
Robotics has made incredible strides over the past decade, evolving from rigid, pre-programmed machines into intelligent systems capable of dynamic decision-making. From simple robotic arms used in Tesla’s gigafactories to NASA’s rovers exploring Mars, robotics has increasingly relied on advancements in artificial intelligence (AI) to tackle complex, real-world challenges.
Reach out to discuss this topic in depth.
Robots are no longer just tools; they have become collaborators in our daily lives. These advancements have propelled robotics beyond mere task execution, enabling them to learn, adapt, and innovate. For example, OpenAI’s Dactyl taught a robotic hand to solve a Rubik’s Cube using reinforcement learning, showcasing how AI can master tasks through trial and error in simulated environments.
At the core of this evolution is physical AI, a paradigm that enables robots to perceive, interact with, and adapt to the physical world. While Generative AI (gen AI) revolutionized content creation and data generation, physical AI is transforming how machines sense, learn, and respond to real-world complexities.
Unlike traditional robotics, physical AI equips machines with the ability to sense their surroundings, learn from them, and act accordingly to perform tasks such as navigating across obstacles or assisting in surgeries.
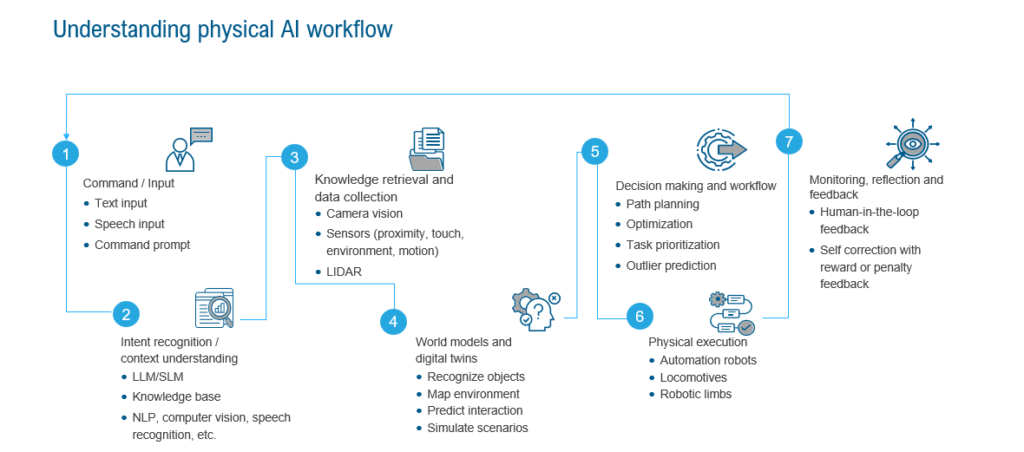
The brain behind the bots: world models and digital twins
Central to the transformation of robotics is the emergence of world models, a groundbreaking approach that enables robots to simulate and predict real-world scenarios. Much like Doctor Strange in Avengers: Infinity War exploring 14 million outcomes to find the one path to victory, world models allow machines to anticipate the trajectory of moving objects, plan optimal paths, and adapt to unforeseen changes. Similar to how gen AI runs on Large Language Models (LLMs), physical AI runs on the foundation of world models.
Unlike LLMs, which excel at processing and generating text based on linguistic patterns, world models are specifically designed to perceive, understand, and navigate physical environments. While LLMs rely on textual data to generate summaries and analyze sentiment, world models process sensor inputs such as vision, depth perception, and motion tracking. This enables robots to think ahead, generate action tokens, and navigate the physical world with precision.
To further enhance the capabilities of world models, digital twins act as a tool that bridges the gap between virtual simulations and real-world deployment. Digital twins enhance world models by providing a virtual testing ground that simulates real-world conditions, improving predictive accuracy, operational efficiency, and decision-making before deployment.
For instance, NVIDIA’s Omniverse provides physics-based simulations for robotics, while Siemens Digital Twin enables industrial automation testing before real-world implementation. Together, world models and digital twins pave the way for autonomous systems that can learn, adapt, and optimize their actions before they ever interact with the real world.
From labs to life: real world use cases of physical AI
The integration of Physical AI, world models, and digital twins is transforming industries such as healthcare, warehousing, agriculture, and transportation, bringing innovation from labs to life. For example:
Healthcare:
Robotic systems like Intuitive Surgical’s da Vinci System leverage digital twins to simulate surgeries, enabling precision and reducing risks.
Warehousing:
Amazon Robotics uses world models and digital twins to optimize inventory movement and navigate complex layouts, enhancing order fulfillment efficiency.
Agriculture:
John Deere’s autonomous tractors employ Physical AI and crop-specific digital twins to predict field conditions, optimize planting strategies, and increase yields while conserving resources.
Transportation:
Waymo’s self-driving vehicles integrate road and traffic digital twins with Physical AI to simulate driving scenarios, improving safety and reliability.
These applications showcase how these advanced technologies are solving real-world challenges, driving efficiency, and reshaping industries globally.
Apart from mainstream industrial applications, companies are pioneering innovative use cases for world models, pushing the boundaries of what robots can achieve. For instance, IntBot, an early adopter of NVIDIA COSMOS, is training its robots to exhibit empathy through world models.
By leveraging synthetic data, these robots can simulate and understand human emotions, enabling them to provide meaningful companionship and engagement. Through natural conversations and gestures, IntBot’s robots adapt to users’ emotional states, offering personalized interactions that go beyond task execution.
Collaborative synergy: the driving forces behind physical AI
Behind every successful deployment lies a robust ecosystem of providers, each contributing a critical piece to the puzzle.
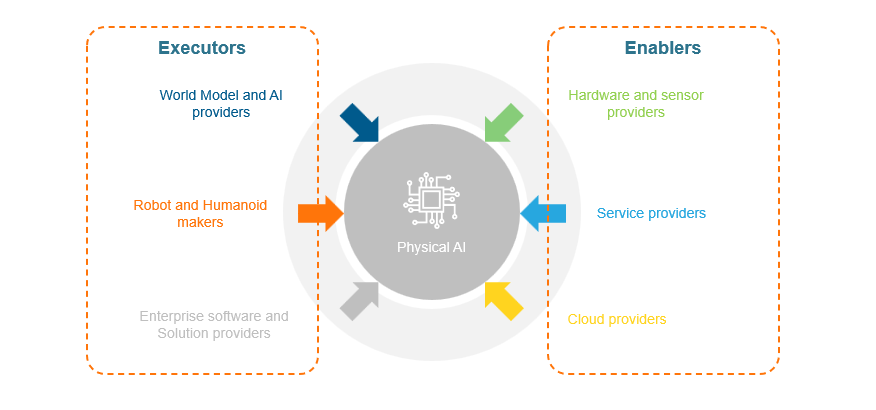
Robot and humanoid makers such as Boston dynamics and Agility are designing the physical systems that interact with and adapt to the world around them. These machines rely on the intelligence provided by world model and AI providers, who create the cognitive frameworks that allow robots to simulate, predict, and make decisions in real time. Supporting these systems, hardware and sensor makers supply essential components like Light Detection and Ranging (LiDAR), cameras, and processors, enabling robots to perceive and act with precision.
Meanwhile, enterprise software and solution providers integrate these elements into tailored solutions for industries, leveraging cloud providers for the computational power needed to process data, train models, and enable real-time operations. Finally, service providers ensure the smooth deployment, operation, and maintenance of these technologies, bridging the gap between innovation and practical application. This interconnected ecosystem is the backbone of the physical AI revolution, transforming groundbreaking ideas into scalable, real-world solutions.
The way ahead
The next phase of Physical AI will see robots becoming more autonomous, predictive, and seamlessly integrated into real-world environments. With advancements in world models, digital twins, and real-time learning, robots will significantly enhance their ability to adapt, collaborate, and optimize decision-making across industries such as healthcare, logistics, and urban mobility.
This progression will enable them to transition from early-stage applications into fully integrated, large-scale partners in the workforce. However, addressing challenges in AI ethics, security, and human-machine collaboration will be crucial for responsible deployment.
Across industries, robots are transitioning from mere tools to indispensable partners, reshaping the boundaries of human-machine collaboration. As Physical AI continues to evolve, it will unlock possibilities once confined to science fiction, driving innovation and efficiency across the global landscape.
If you found this blog interesting, check out our GTC 2025: Did Jensen Just Bring Physical AI Forward? | Blog – Everest Group, which delves deeper into another topic regarding AI.
To explore how physical AI can help your organization successfully navigate future transformation, contact Abhiram Srivatsa ([email protected]) and Samikshya Meher ([email protected]).